Over the last 3 months, I’ve received four new Amazon Alexa devices – one Echo Dot (from re:Invent) 1 and an Echo, a Dot, and a Tap from Amazon and Hackster for my winning entry in their API contest. That, plus the Echo I already owned, my wife’s fire tablet, my two Amazon Fire TVs, and my custom Mystic Mirror give me a total of 9 Alexa-enable devices in my home!!!
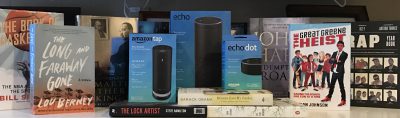
With all those devices, it was natural for me to accelerate my adoption of the Alexa in the house, with a focus on smart lights.
I spent a few weeks researching options. In short: there are a lot of products out there. I knew that I wanted to control 3 “bays” of lights:
- Family room recessed lights (6) – BR40
- Kitchen recessed lights (4) – BR40
- Outside porch/front door lights (2) – standard A19
Given the number of lights, I felt that the most cost-effective solution was to go with smart switches. [Note: the added benefit of smart switches was that I wanted to control the lights by voice and wall switch, which a smart bulb would not let me do].
From there, I narrowed my choices to Z-wave switches (partnered with a Samsung SmartHub or Wink) or Lutron Caseta system. I liked the flexibility of Wink or SmartHub (it can support multiple technologies), but I ultimately went with the Caseta. I read multiple reviews about how great the Caseta system worked… and with a smart home skeptic in the house, I wanted to make sure that I implemented something that would be responsive and easy to use.
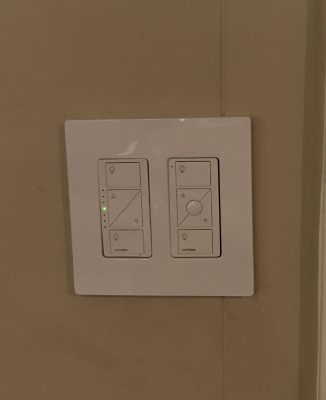
I’ve only had the system in two days, but so far, I’m beyond elated about my choice. Other systems might be slightly less expensive…. but this just WORKS. Plus, there are some added benefits:
- I’ve added dimming functionality (which is a big deal at 2AM in the morning).
- I’ve scheduled my porch lights to turn on at sunset and off at 11pm – so, even less work for me there.
- I’m starting to win my wife over (in regards to using voice commands to control our home).